Improved Target Identification
Machine learning algorithms have revolutionized target identification in drug discovery by analyzing vast amounts of data to identify potential drug targets with high accuracy, prioritizing targets based on various criteria, and even discovering new targets that may have been overlooked.
Accelerated Compound Screening
Machine learning has significantly accelerated compound screening through virtual screening and high throughput screening. By analyzing data and predicting compound activity, machine learning algorithms can quickly prioritize compounds for further testing, saving time and resources in the drug discovery process.
Predictive Toxicology Assessment
Machine learning enables predictive toxicology assessment, predicting the toxicological properties of compounds with high accuracy. By analyzing relationships between chemical structures and toxicological outcomes, these algorithms can reduce the need for animal testing and expedite drug discovery.
Enhanced Drug Design and Optimization
Machine learning algorithms optimize drug design by leveraging pharmacokinetic modeling and virtual screening techniques. This allows for improved understanding of drug interactions in the body, faster identification of potential drug candidates, and more personalized treatment options.
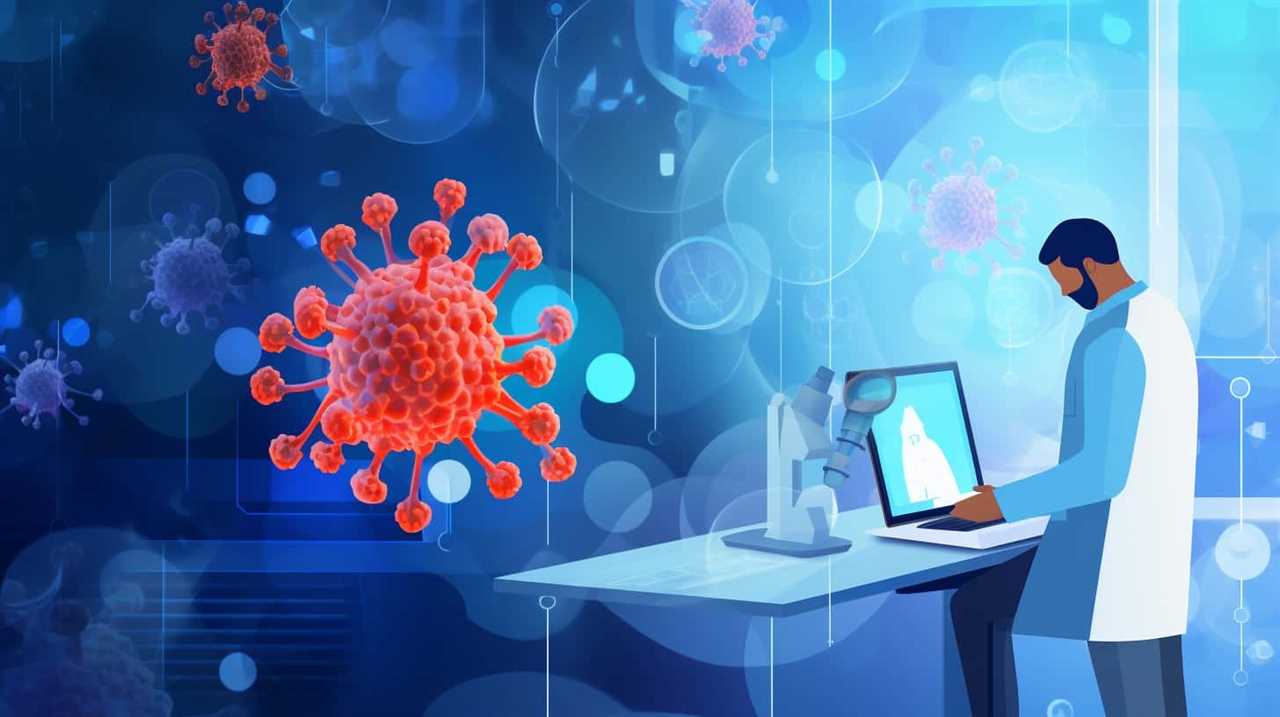
Development of Personalized Medicine
Machine learning in medical AI is transforming personalized medicine by tailoring treatments to individual patients based on their genetic makeup and characteristics. By analyzing large datasets, machine learning algorithms can identify personalized treatment options, maximizing effectiveness and minimizing adverse effects.